Products

Report | August 2023
A Machine Learning Evaluation Framework for Place-based Algorithmic Patrol Management
American law enforcement agencies are increasingly adopting data-driven technologies to combat crime, with the market for such technologies projected to grow significantly in the coming years. One prevalent approach, place-based algorithmic patrol management (PAPM), analyzes data on past crimes to optimize police patrols. These systems promise several benefits, including efficient resource allocation, reduced bias, and increased transparency. However, the adoption of these technologies has raised ethical and social concerns, particularly around privacy, bias, and community impact. This report aims to provide a comprehensive framework, including many concrete recommendations, for the ethical and responsible development and deployment of PAPM systems. Targeting developers, law enforcement agencies, policymakers, and community advocates, the recommendations emphasize collaboration among these stakeholders to address the complex challenges presented by PAPM. We suggest that failure to meet the proposed ethical guidelines might make the use of such technologies unacceptable. This report has been supported by National Science Foundation awards #1917707 and #1917712 and the Center for Advancing Safety of Machine Intelligence (CASMI).

Pre-print | January 2024
A debiasing technique for place-based algorithmic patrol management
In recent years, there has been a revolution in data-driven policing. With that has come scrutiny on how bias in historical data affects algorithmic decision making. In this exploratory work, we introduce a debiasing technique for place-based algorithmic patrol management systems. We show that the technique efficiently eliminates racially biased features while retaining high accuracy in the models. Finally, we provide a lengthy list of potential future research in the realm of fairness and data-driven policing which this work uncovered.
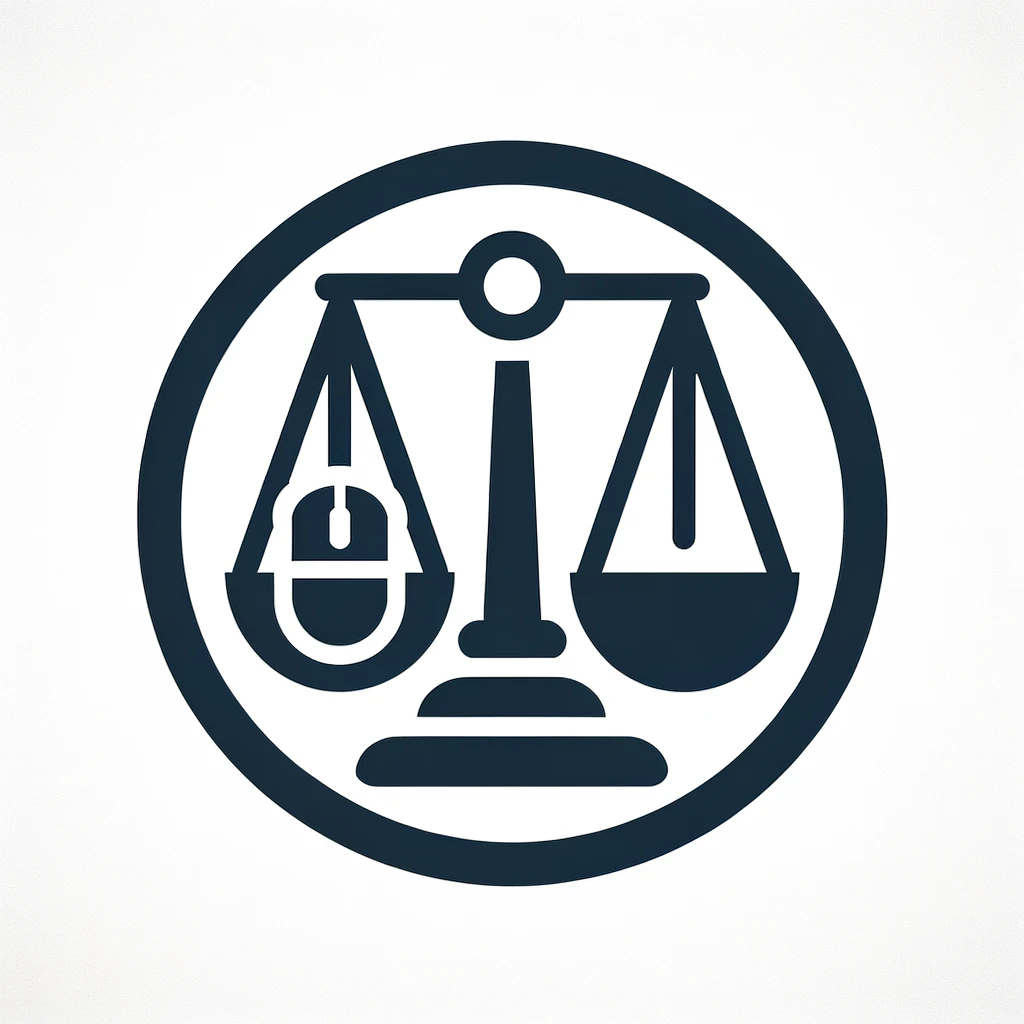
Syllabus | December 2023
Police Ethics and Police Technology
The first professional police force was founded in Boston, Massachusetts in 1838. Since that time, police have long played a central role in the promotion of safety and security in the United States. But a growing chorus of critics is questioning the place of policing in maintaining social order. Among the concerns being voiced are that police work increasingly violates civil liberties, that policing is racially biased in ways that oppress marginalized people, and that the scope of police work outstrips their expertise. Emerging policing technologies can either ameliorate or aggravate these concerns. In this course, we will investigate, through the methods of moral philosophy, the moral foundations of policing, some recent ethical controversies about the role and conduct of police in society, and the appropriate role of technology in policing. Topics include an introduction to ethical issues in artificial intelligence, the role of police in society, institutional critiques of policing and big data technology, police discretion, predictive policing, surveillance and data collection, non-lethal weapons and police use of force, and future directions in policing and policing technology.

Article | June 2023
What’s Wrong with Predictive Policing?
As the European Union prepares to vote on the Artificial Intelligence Act, which aims to regulate AI applications based on their risk levels, the inclusion of predictive policing systems on the list of banned technologies has sparked debate. Predictive policing, particularly the place-based variety, uses algorithms to identify high-risk locations for crime, aiding law enforcement in resource allocation. However, critics argue that such systems perpetuate racial biases and result in a "runaway feedback loop" of escalating police attention in minority communities. The article delves into the ethical and empirical complexities of predictive policing, questioning the fairness of additional police attention and suggesting community involvement as a potential solution. While the EU Act treats all predictive policing as high-risk, the nuanced ethical landscape of these systems calls for a more differentiated regulatory approach, urging policymakers to carefully weigh both societal benefits and ethical risks.

Journal Article | April 2023
Should Algorithms that Predict Recidivism Have Access to Race?
Recent studies have shown that recidivism scoring algorithms like COMPAS have significant racial bias: Black defendants are roughly twice as likely as white defendants to be mistakenly classified as medium- or high-risk. This has led some to call for abolishing COMPAS. But many others have argued that algorithms should instead be given access to a defendant's race, which, perhaps counterintuitively, is likely to improve outcomes. This approach can involve either establishing race-sensitive risk thresholds, or distinct racial ‘tracks’. Is there a moral difference between these two approaches? We first consider Deborah Hellman's view that the use of distinct racial tracks (but not distinct thresholds) does not constitute disparate treatment since the effects on individuals are indirect and does not rely on a racial generalization. We argue that this is mistaken: the use of different racial tracks seems both to have direct effects on and to rely on a racial generalization. We then offer an alternative understanding of the distinction between these two approaches—namely, that the use of different cut points is to the counterfactual comparative disadvantage, ex ante, of all white defendants, while the use of different racial tracks can in principle be to the advantage of all groups, though some defendants in both groups will fare worse. Does this mean that the use of cut points is impermissible? Ultimately, we argue, while there are reasons to be skeptical of the use of distinct cut points, it is an open question whether these reasons suffice to make a difference to their moral permissibility.

Journal Article | April 2022
Public Trust, Institutional Legitimacy, and the Use of Algorithms in Criminal Justice
A common criticism of the use of algorithms in criminal justice is that algorithms and their determinations are in some sense “opaque”—that is, difficult or impossible to understand, whether because of their complexity or because of intellectual property protections. Scholars have noted some key problems with opacity, including that opacity can mask unfair treatment and threaten public accountability. In this paper, we explore a different but related concern with algorithmic opacity, which centers on the role of public trust in grounding the legitimacy of criminal justice institutions. We argue that algorithmic opacity threatens the trustworthiness of criminal justice institutions, which in turn threatens their legitimacy. We first offer an account of institutional trustworthiness before showing how opacity threatens to undermine an institution's trustworthiness. We then explore how threats to trustworthiness affect institutional legitimacy. Finally, we offer some policy recommendations to mitigate the threat to trustworthiness posed by the opacity problem.
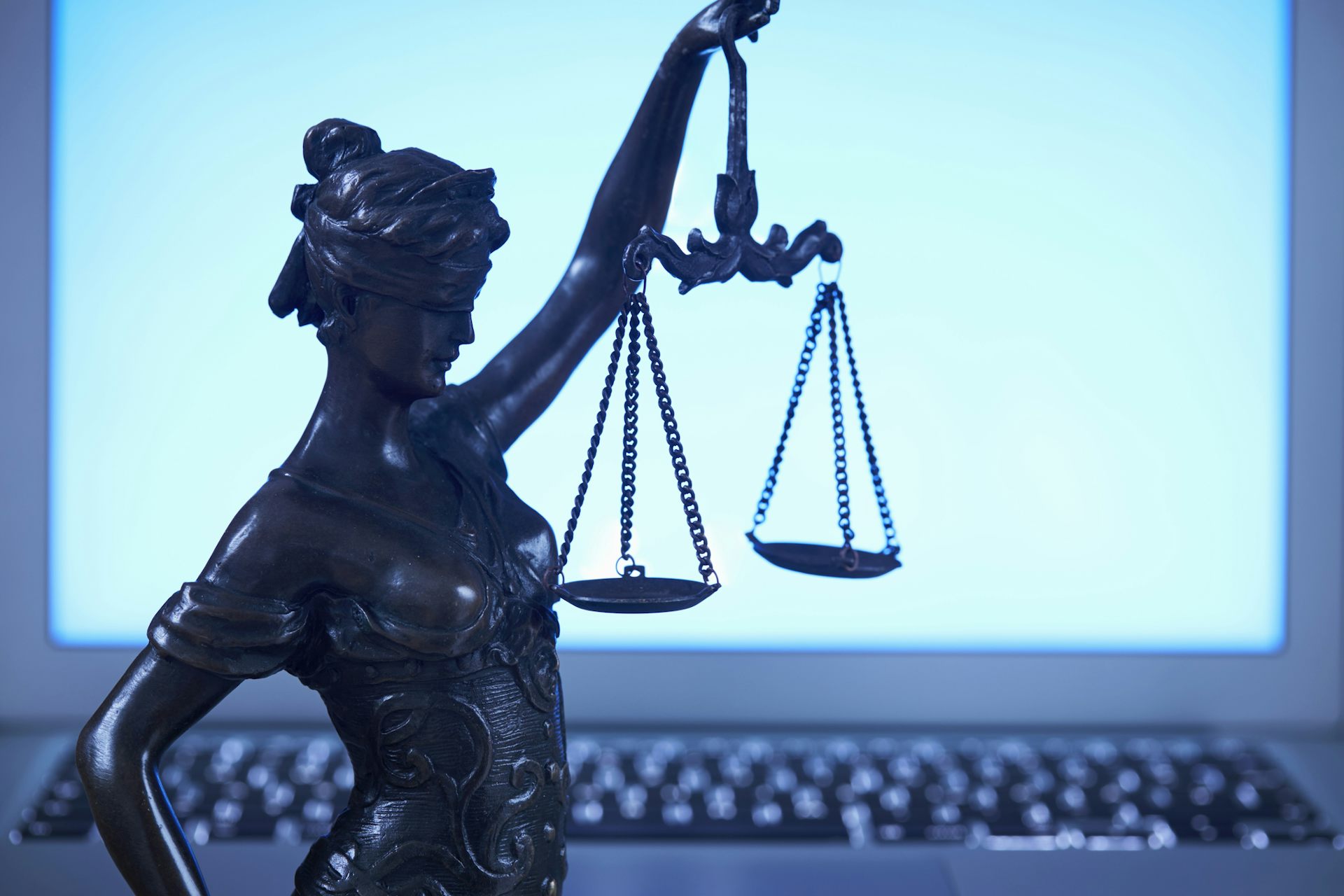
Article | March 2022
Criminal justice algorithms: Being race-neutral doesn’t mean race-blind
The First Step Act, passed in 2018, aimed to reform the U.S. criminal justice system, offering early release to low-risk federal inmates identified through an algorithm called PATTERN. While initially celebrated, a Department of Justice review found that PATTERN disproportionately overpredicts recidivism rates among minority inmates, inadvertently perpetuating racial biases. Ethical and legal debates now center on whether incorporating racial variables into the algorithm can make it more equitable without violating constitutional principles. Duncan Purves argues that such a change could improve the system's accuracy across all racial groups without making it a zero-sum game, thereby advancing justice without compromising public safety. This paradox raises critical questions about the role of 'race blindness' in achieving genuine racial equality.
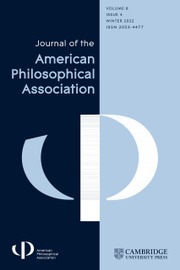
Journal Article | March 2022
Fairness in Algorithmic Policing
This paper argues that the prevailing focus on racial bias has overshadowed two normative factors that are essential to a full assessment of the moral permissibility of predictive policing: fairness in the social distribution of the benefits and burdens of policing as well as the distinctive role of consent in determining fair distribution. When these normative factors are given their due attention, several requirements emerge for the fair implementation of predictive policing. Among these requirements are that police departments inform and solicit buy-in from affected communities about strategic decision-making and that departments favor non-enforcement-oriented interventions.
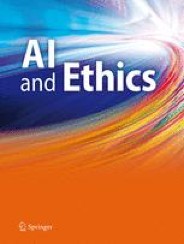
Journal Article | January 2022
Five ethical challenges facing data-driven policing
This paper synthesizes scholarship from several academic disciplines to identify and analyze five major ethical challenges facing data-driven policing. Because the term “data-driven policing” encompasses a broad swath of technologies, we first outline several data-driven policing initiatives currently in use in the United States. We then lay out the five ethical challenges. Certain of these challenges have received considerable attention already, while others have been largely overlooked. In many cases, the challenges have been articulated in the context of related discussions, but their distinctively ethical dimensions have not been explored in much detail. Our goal here is to articulate and clarify these ethical challenges, while also highlighting areas where these issues intersect and overlap. Ultimately, responsible data-driven policing requires collaboration between communities, academics, technology developers, police departments, and policy makers to confront and address these challenges. And as we will see, it may also require critically reexamining the role and value of police in society.
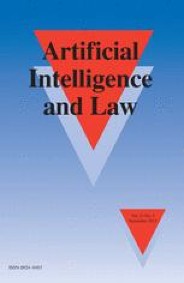
Literature review | April 2021
A review of predictive policing from the perspective of fairness
Machine Learning has become a popular tool in a variety of applications in criminal justice, including sentencing and policing. Media has brought attention to the possibility of predictive policing systems causing disparate impacts and exacerbating social injustices. However, there is little academic research on the importance of fairness in machine learning applications in policing. Although prior research has shown that machine learning models can handle some tasks efficiently, they are susceptible to replicating systemic bias of previous human decision-makers. While there is much research on fair machine learning in general, there is a need to investigate fair machine learning techniques as they pertain to the predictive policing. Therefore, we evaluate the existing publications in the field of fairness in machine learning and predictive policing to arrive at a set of standards for fair predictive policing. We also review the evaluations of ML applications in the area of criminal justice and potential techniques to improve these technologies going forward. We urge that the growing literature on fairness in ML be brought into conversation with the legal and social science concerns being raised about predictive policing. Lastly, in any area, including predictive policing, the pros and cons of the technology need to be evaluated holistically to determine whether and how the technology should be used in policing.
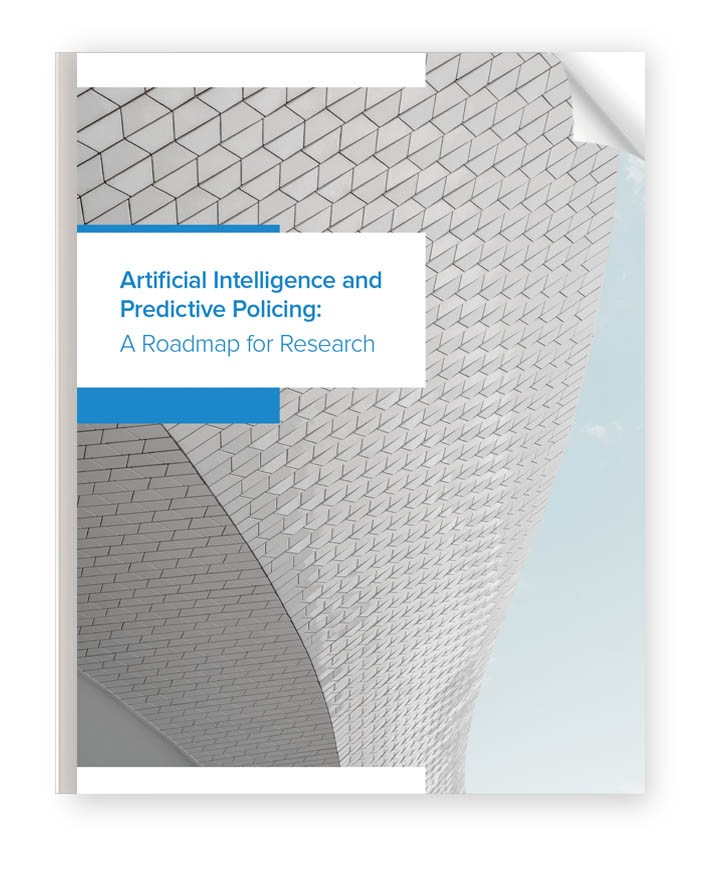
Report | September 2020
Artificial Intelligence Ethics and Predictive Policing: A Roadmap for Research
Against a backdrop of historic unrest and criticism, the institution of policing is at an inflection point. Policing practices, and the police use of technology, are under heightened scrutiny. One of the most prominent and controversial of these practices centrally involves technology and is often called "predictive policing." Predictive policing is the use of computer algorithms to forecast when and where crimes will take place — and sometimes even to predict the identities of perpetrators or victims. Criticisms of predictive policing combine worries about artificial intelligence and bias, about power structures and democratic accountability, about the responsibilities of private tech companies selling the software, and about the fundamental relationship between state and citizen. In this report, we present the initial findings from a three-year project to investigate the ethical implications of predictive policing and develop ethically-sensitive and empirically-informed best practices for both those developing these technologies and the police departments using them.
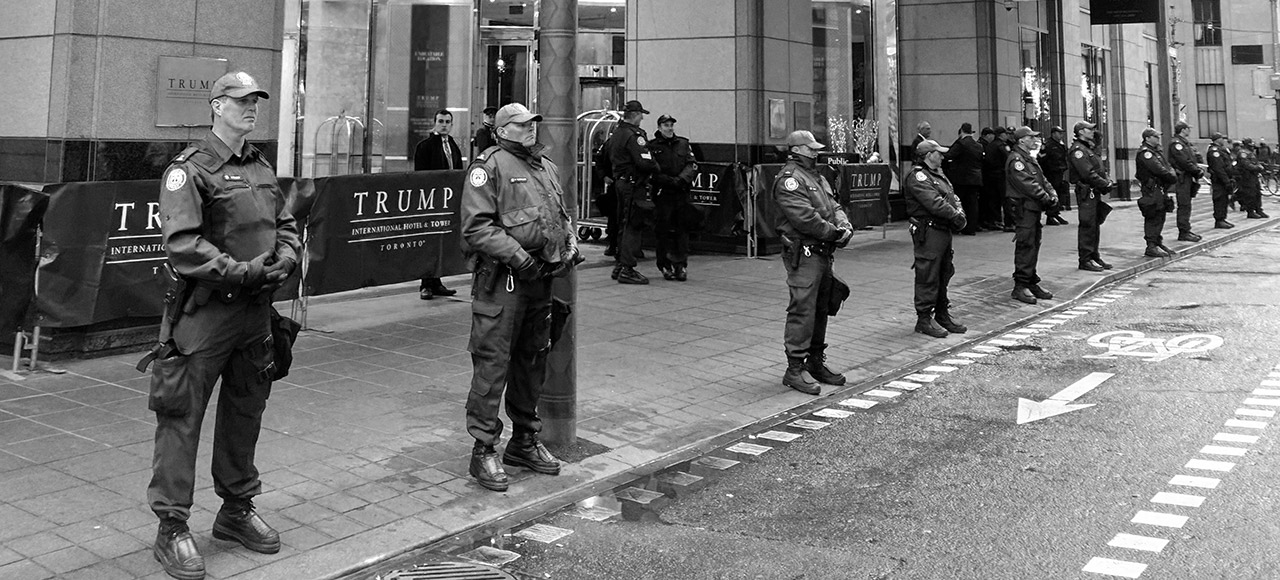
Article | June 2, 2020
Winning the Battle, Losing the War
At least since the Industrial Revolution, humanity has had a troubled relationship with technology. Even while standards of living have skyrocketed and life expectancies lengthened, we have often been shocked or dismayed by the unforeseen disruptions that technology brings with it. I suspect that a major source of this myopia is the naivete of one popular view of technology: that technologies are merely neutral tools and that our engagements with particular technologies are episodic or, in the words of Langdon Winner, brief, voluntary, and unproblematic. I think view is simplistic – and appreciating both the consequences of longer-term technological policies and the interplay between a technology and its social context can help us anticipate these negative consequences. In particular, we should appreciate how even an efficient and reliable technology can nurture social circumstances that will undermine the very goals that technology is meant to serve.